The beautiful city of Sydney hosted the 8th international conference of Learning Analytics 2018 (LAK'18). There with my colleagues of OUNL and DIPF, we brought our contributions to the research community.
This year my main contribution constituted in the organisation of Multimodal Data Challange at the Learning Analytics Hackathon. Together with my colleagues Jan Schneider (DIPF) we brought our approach to capturing, storing, analysing, annotating and exploiting multimodal data. The challenge was introduced by the info document of the Hackathon, as well as the 3-pages submission for the LAKHackathon submission Multimodal challenge: analytics beyond user-computer interaction data.
In addition, to inform the researchers who work in the multimodal learning analytics field (CrossMMLA group) we also submitted a paper The Big Five: Addressing Recurrent Multimodal Learning Data Challenges, to appear in the CEUR conference proceedings. This contribution further explained the approach proposed for handling multimodal data, including a description of our main prototype, the Learning Hub.
Now me and @SuperHeroBooth presenting out our paper "The Big Five: addressing recurrent multimodal learning data challenges" at the #CrossMMLA workshop at #LAK18 #lakhackathon #multimodalhack Paper available here https://t.co/e3wbFCrSOZ pic.twitter.com/CMOmxrDbnJ
— Daniele Di Mitri ⏩️🦣 (@dimstudi0) March 6, 2018
Hackathon Takeaways
But was the Hackathon useful? Short answer: YES, it contributed expanding the horizon of our framework.
The first insight we got is the need to extend our framework also to classroom activities. The approach we initially had in mind was designed for recording practical working experience for psychomotor skills training, i.e. trainees at school or at workplace need to learn certain practical skills. In these settings, the learner needs to be equipped with several sensors. This idea stems from the setup used in the WEKIT project.
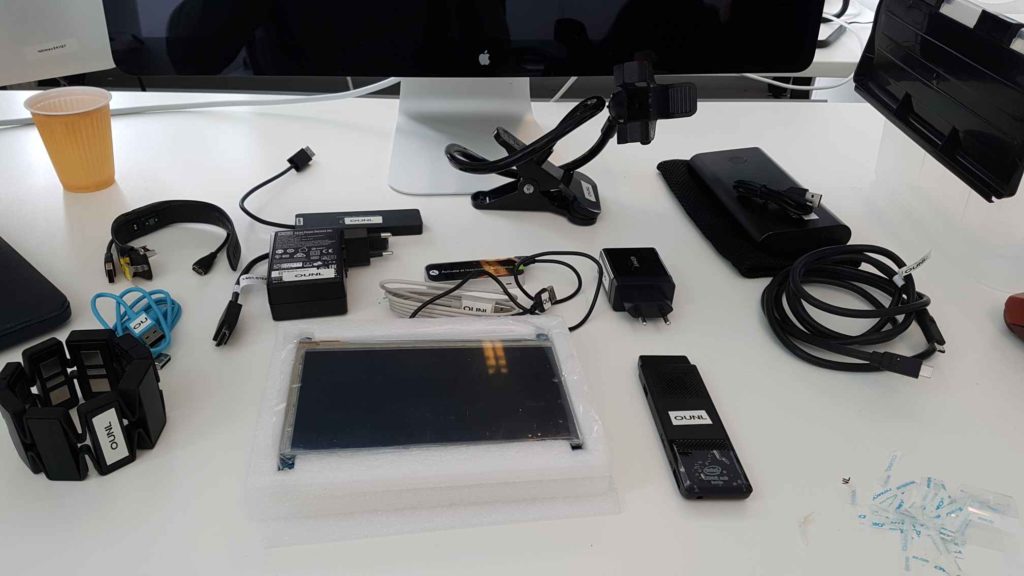
However is sensible to guess that in typical face-to-face classroom interactions, learners cannot always be equipped with a full sensor suite. One idea which came out from the hackathon is to develop a mobile application to work in conjunction with our framework. This app has two purposes: 1) track the available sensor data and push it to LearningHub; 2) get self-reported questionnaires from the learner.
This app could work according to the Timetable downloaded from the school server and remind the student where is he/she supposed to be. The data gathered by the system will be processed and fed back to the teacher in form of analytics dashboard.
It could also monitor also classroom attendance and provide valuable data to the teachers and to the school principals on how to best allocate room space.
Another insight and the potential requirement for the framework which came out from Hackathon is the possibility to provide real-time annotations from external judges. This would complement the retrospective annotations of both experts (using the Visual Inspection Tool) and by the learner using the self-reporting tool embedded in the app.
Finally, one big feature we realised is missing in our architecture is a system for mapping the multimodal data to some sort of user authentication. Up until now, we have been thinking only in terms of wearable, one-to-n technologies: i.e. one learner will generate from one to multiple datasets. We have not been taking into account the n-to-n scenarios, in which multiple learners generate multiple datasets. This would require some sort of user-authentication engine to recognise one potential learner in the recording.
However, the two days workshop were not enough to explore the complexity of the challenges here described. Some research questions remained unsolved. We are still wondering what is the best approach for integrating the sensor data captured in the multimodal data store via the LearningHub with the user interaction data that are typically expressed with Experience API.
XAPI is a well-known standard to specify the learner-centred experiences. A constraint that XAPI has is in working with multimodal data since the learner-actions are not clearly defined or can be hidden in the data. For this reason, the architecture needs some kind of action-recognition layer. This could be trained with the expert annotations.
Next steps
Creating a technological framework that which support all the nuances of learning with multimodal data can be quite complex. We are planning to continue our effort in doing so by continuing the development of the Learning Hub and the Visual Annotation Tool. We will be probably joining the Dutch Hackathon to be organised within LSAC 2018.
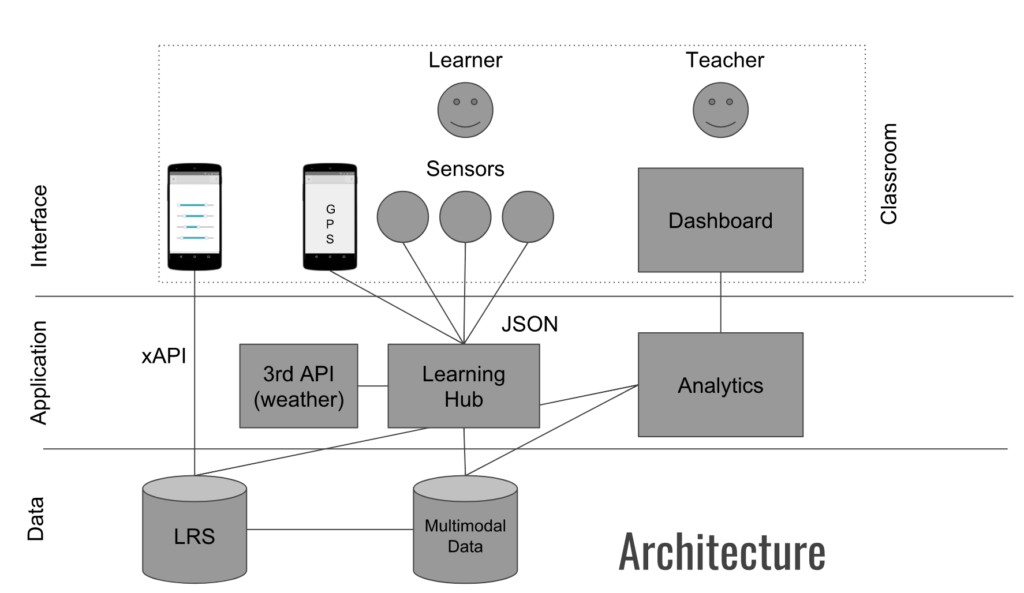