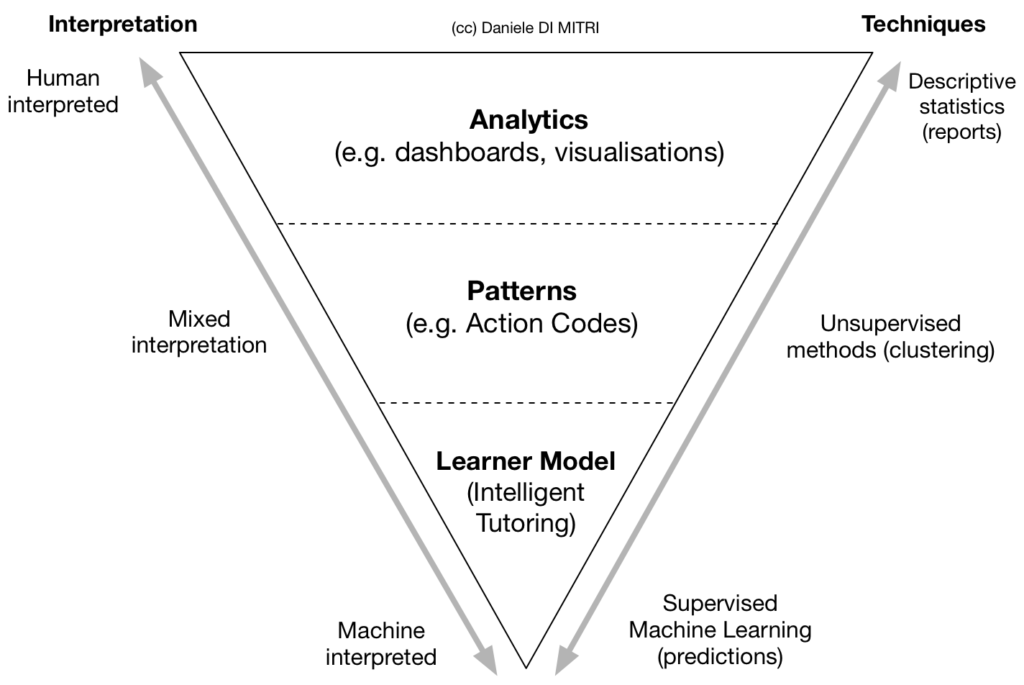
Originally posted in Lilab.eu
Last week the European Conference on Technology Enhanced Learning (#ECTEL2017) took place in Tallinn University with an overarching theme: "data-driven approaches in education". This year's conference focused on the role of data and learning analytics as means for deciphering and improving learning and teaching practices.
This year, also in ECTEL17 great focus was given to multimodal data, i.e. the data sources like as sensors and wearables which aim to capture the learning behavior in the offline physical space, rather than in the online virtual environments. For instance, a new workshop was initiated merging two existing ones: the Cross Multimodal Learning Analytics Workshop (crossmmla.org). In a previous article, I pointed out how multimodality was also of great focus of the Learning Analytics conference. There is hence great motivation in using technologies like eye-tracking, heart-rate trackers, brain waves sensors to monitor the aspects of learning which can seamlessly be captured by sensors.
Nevertheless, the feeling I had after attending a one-day workshop, several presentations, one project meeting (WEKIT) and a lot of other informal talks with researchers in the field is that there is great confusion around HOW the data should be used. There seems to be a tendency of being fascinated by technologies like "sensors" and "analytics". This was striking, for example, the best paper nominee Jivet et al by my colleague Ioana, who concluded that educational concepts are very rarely supported in learning analytics dashboards.
At ECTEL I was asked to make an overview of how Learning Analytics could be used for Multimodal Data so I decided to come up with a simple pyramid which summarises the use of Multimodal Data. The Pyramid is divided into three levels:
- The topmost is the purpose of ANALYTICS. This purpose is the most recurrent and accessible. It uses information visualisation techniques which are usually grouped into Dashboards. The techniques used are descriptive statistics: meaning reporting back to the user the historical development of the monitored dimensions. It relies on the human looking at the visualisations and deriving meaning out of them.
- The middle purpose is the PATTERNS. This term is wrongly used as an all-encompassing term. Patterns, however, means looking at recurrent codes (action codes) that happen into data. The difference with the previous analytics is that more automated methods are used such as unsupervised methods like clustering. This is a mixed interpretation model since it both relies on human making a good number of clusters decision but also on computer methods to actually perform the clustering.
- The third purpose is the LEARNER MODEL. This is the highest and the most complex aim for multimodal data: i.e. modelling the learner to allow personalisation and adaptation. Modelling the learner automatically would allow the machine to be able to adapt teaching strategies to each individual learner. For this reason, the learner model is the base of the Intelligent Tutoring Systems. This purpose requires supervised machine learning, meaning that it requires experts to annotate with labels the learning experience which will be later used to train the models. A classic example for this is the affect detection: experts are asked to pair the learner's multimodal data with their emotions (say every 20 seconds) and based on this labelling computational models are trained to be able to predict emotions from new data.